On death and dying
Or "The epistemological problems with 'Statistical and numerical errors made by the US Centers for Disease Control and Prevention During the COVID-19 Pandemic' by Krohnert et. al."
Abstract
A recent paper (“Statistical and numerical errors made by the US Centers for Disease Control and Prevention During the COVID-19 Pandemic”) makes the argument that the disease impact of the SARS-CoV-2 virus (“SARS2”), particularly in children, has been systematically exaggerated by the US Centers for Disease Control (CDC).
With the implication that these errors are not mere errors but are rather deliberate attempts to mislead the public about the impacts of SARS2 for the explicit purpose of imposing nefarious policy objectives. However the paper never addresses what these policy objectives might be1.
The paper’s conclusions are based on what can only charitably be called profound misunderstandings of the nature of disease and death as well as the structure and limitations of public health bureaucracies in the United States. Less charitably it can be said that the paper’s conclusions are explicit disinformation deliberately manufactured to undermine collective public health measures in furtherance of a right-wing laissez-faire ideology that is intolerant of the democratic ideals characteristic of liberal democracies such as ours.
At risk are effective future public health measures to control the pathological impact of disease — not only in the United States but in the entire World — should the reasonable-sounding conclusions of the paper be uncritically accepted at face value.
Part One: On Life and Death
We do not know what it means to be alive. We say with confidence that a rock is not “alive” but a cockroach is “alive.” Yet if pressed to describe the fundamental difference between a rock and a cockroach that makes one not living and the other living, no one can precisely say.
We can list out properties that living things have such as mobility, chemical reactions involving catalysts (“enzymes”), respiration, sentience, etc. But inevitably we can find examples of things that we clearly believe to be alive that do not possess one or more of those properties. And we can list examples of things we do not consider to be alive that do exhibit some or all of those properties.
Because it is impossible to define what is and what is not alive, it’s difficult to define when something transitions from being not alive to being alive. Or to transition from being alive to being dead.
Is an egg alive? Or does it become alive once fertilized? Or perhaps it becomes alive once a sufficient amount of cell division occurs? When does a human die? When their respiration stops? When their brain activity stops?
We don’t know, precisely. Because of that imprecision, questions about what it means to be alive and what it means to be dead are unanswerable by science, by medicine and certainly by bureaucracies. Instead we leave those questions to debate by philosophers while the rest of us muddle along lining up behind the philosopher whose viewpoints most closely align with our ideological beliefs.
I spent over two decades in health care, particularly in the subdomain of health care data related to clinical presentations, long-term (chronic) care management and methods of collection, curation and presentation of health care data. In those two decades I founded two separate companies whose explicit mission was to produce tools and procedures to improve the state of health care data. I also served as a director of healthcare initiatives, developing patient/provider communication tools, at a very large consultancy.
The lesson that I learned in those decades is that health care data is unbelievably messy, unhygienic, inaccurate and incomplete.
It is a complete trainwreck. Always has been and given our inability to answer even fundamental questions such as “is it dead or alive?” will very likely remain that way for some time to come. It makes us uncomfortable to admit it (which is why we don’t) but our understanding of life, biology and health is still in its infancy.
No Ph.D rocket scientist in the World would be able to earn a living if they knew as little about how rockets actually work as the average Ph.D immunologist or Ph.D virologist knows how the human immune system or viruses actually work.
It’s no surprise that the data is a wreck given that we don’t even know what we’re looking at.
Why did it die?
Well-funded market campaigns by large health care organizations as well as depictions in popular media are designed to create an impression in the public’s mind of a hyper-competent medical industry. Think of the gleaming television ads and highway billboards proclaiming daily miracles from organizations like the Mayo Clinic, Cleveland Clinic, St. Judes, etc.
Or popular television shows like “House,” “St. Elsewhere,” “Quincy, M.E.” etc. where each episode ends not with a discussion of why the insurance company denied payment for a particular procedure (and the patient died) but instead how at the moment of inflection there was a “Eureka!” moment. Giving the impression that no one ever dies of an idiopathic (“unknown”) cause which means that Quincy the M.E. never has a busy day in his life.
That’s not how it is in the real world. In the real world, for anything other than the most obvious reason, medicine has an extremely hard time diagnosing the “root cause” of illness and instead has to resort to describing illness through a thesaurus of synonyms for “no f*cking idea.” Words like “idiopathic,” “rheumatic,” “ischemic,”“syndrome,” etc. all of which really just mean “no f*cking idea” without the embarrassment to well-compensated professionals of having to actually say “I have no f*cking idea.”
Because medicine cannot identify the root cause of illness in the first place, it is also extremely imprecise in assigning a root cause of death. The word doing the heavy lifting here is the word “syndrome.”
A syndrome is simply the name of a set of symptoms that are associated with a particular set of deaths. It’s a way not of telling you why a person died but that, when they did die, it looked very similar to the deaths of this other set of other people who also died.
For example, SIDS is the name for “Sudden Infant Death Syndrome.” It means a child who dies for reasons we don’t exactly know but they died young and usually were discovered dead in a bed or a crib by a parent or caregiver. If a death certificate says a child died of SIDS it doesn’t tell you why they died. It just tells you they were a child and the cause of actual death is a total mystery. Case closed.
We have other examples. One of my favorites is the umbrella term “Influenza-LIKE-illness” or “ILI.” An ILI is basically any illness that presents with symptoms of respiratory distress such as coughing, sneezing, pneumonia, etc. And other general symptoms such as fever, fatigue, etc.
The problem with that is that virtually all illnesses, from heart failure to broken bones to cancer will produce symptoms of respiratory distress, fatigue, etc. as those symptoms are products of our body’s innate immune system that are triggered whenever the body feels itself to be in some form of distress.
My late wife died of an autoimmune disease yet all the way to her autopsy doctors assured her that she just had “the flu” based on nothing more than the clinical manifestations of her symptoms.
We don’t know why it died
The exact reason for why a person has been hospitalized or why a person died is extremely difficult to determine in all but the most obvious cases (railroad spike through the head?). Most illnesses present with nearly identical “flu-like” symptoms irrespective of the nature or severity of illness. Indeed some of the most severe illnesses like polio, hepatitis and HIV present with absolutely mild or nonexistent symptoms altogether.
Yet the public again has been conditioned by false narratives of medical hyper-competence and the false narrative that severity of disease is correlated with severity of symptoms. In the real world, the medical establishment trips over itself trying to find out what is going on and the most dangerous diseases usually present with very mild symptoms all the way up to the point of death from the disease.
And we have no way of finding out
The United States is a rough conglomeration of more than fifty largely sovereign entities (the states, territories, Washington D.C.,etc.) — think of it as the European Union with the only difference is that instead of calling California the largest country in the union, it’s the largest state. Beyond that there is little difference.
From a health care perspective what that means is that health care data that happens in California, stays in California. Health care data such as electronic health records, hospital admissions, death certificates, etc. are all generated at the local level (usually the county) and all stay at the local level. What’s more there is absolutely no standardization other than informal and unenforceable guidelines pleading that the way one state records a death should be similar to the way that all other states record deaths.
Unlike, say, the United Kingdom where the entire health care system is owned and operated by the equivalent of the UK’s federal government, the federal government in the US has very little ability to tell the states and counties how to record deaths or how to report deaths to the federal government. Instead the CDC has to request that counties and states voluntarily forward health data to the CDC and then hope that the data from one state will be collected and reported in reasonably the same way it is collected and reported by every other state.
As you can imagine, it doesn’t work out that way. The fact that health care data is created, regulated, collected and disseminated at the local level opens a door to local political differences in health care ideology that conspire to subvert any national ideology with regard to health care.
In other words, if an elected Republican county coroner in Polk county Indiana wants to mark the cause of death on a COVID death certificate as “influenza-like-illness,” instead of COVID, there’s absolutely nothing that anyone can do to stop her and no way for the CDC to know when it finally does receive the death certificate that the death was actually due to a SARS2 infection, not influenza.
Nowhere is this worse than with the case of pediatric (child) deaths. In general it is much more difficult to determine why, exactly, a child dies than it is to determine why, exactly, an adult dies — even though it’s already very hard to determine why an adult dies2.
We have an entire cause of death (SIDS) for children that means nothing more than “we have no idea why the child died and we’re not going to try and find out because it’s too hard.” The only thing close to that for adults is the all-encompassing “dementia” which is understood to mean by everyone as “don’t know why they died but they were old so we don’t have to care.” Luckily in the newest revision of the International Classification of Diseases (ICD) the dementia code has been removed as a valid cause of death.
The precautionary principle
Health information is inherently inexact, incomplete and “dirty.” The root causes of this are:
The difficulties of accurately ascertaining the causes of death.
The nature and relationship of the states to the federal government, particularly with regard to the tenth amendment of the Constitution. The Constitution reserves to the states all sovereign powers not explicitly surrendered to the federal government in the Constitution — including the power to conduct the practice of healthcare as each individual state sees fit.
The utter infancy of many fields such as epidemiology, virology and immunology.
Anyone aware of these structural limitations regarding accurate health care diagnosis perfectly understands that the approach towards a new, out of control, deadly pathogen like SARS-CoV-2 demands an utterly cautious approach. SARS-CoV-2 is a BSL-3 pathogen3 that has a now killed well over twenty million people worldwide and continues to kill over ten thousand Americans every month, including two children every day.
The paper’s authors know all of this this. Which is why when they demand that the CDC produce absolutely exact numbers or else be labelled as “erroneous” they are making demands that they know no organization or institution in the health care field can possibly meet.
The authors know this which is why their demand for perfect accuracy is as hollow as it is cynical and corrosive. Their only purpose is not to improve public health or protect lives. It is to tear down the democratic institutions that we depend on to protect ourselves and our children.
Opportunity knocks
When the public’s expectation of what medical competence should be, based on revenue-generating market campaigns and popular media, collides with boots-on-the-ground realities of a hyper-local balkanized health care data collection, curation and dissemination infrastructure with little to no national standards of data hygiene, it creates opportunities.
Opportunities for cynical exploitation of how the public believes the system works (or should work) vs. the divide of how it actually works. This is the sausage factory that makes our sausage in various flavors of exploitative disinformation such as “with” sausage vs. “from” sausage and “incidental” sausage.
If I am being too obtuse, I am referring to the debates over whether or not someone is hospitalized because of COVID or just with an incidental SARS2 infection.
Or whether they died “from” COVID or just “with” COVID.
These are not debates we have ever had in the past, BTW. No one ever questioned if someone was in the hospital “for” cancer or just “with” cancer. No one ever questioned if someone died “from” AIDS or just “with” AIDS.
No one said to another “your mother didn’t die of influenza, she just died with influenza. She was just old.”
These distinctions between “with” vs. “from” are entirely new. That’s because they were expressly manufactured in order not to clarify and improve the delivery of health care but rather to muddy the waters, sow discord and provide a foundation for withholding care for little more than an ideology of cruelty-for-cruelty’s sake.
And these are intellectually attractive distinctions to anyone who doesn’t understand the constraints actually faced by the system and therefore become easily weaponized by those whose only motivation is to maintain ancient hierarchies of wealth, power and prestige.
Part Two: “Statistical and numerical errors made by the US Centers for Disease Control and Prevention During the COVID-19 Pandemic” as a case study in the above
In their abstract the authors declare:
Background: The Centers for Disease Control and Prevention (CDC) has been a major source of information during the COVID-19 pandemic, guiding policies and practices in many aspects of life. As such, it is imperative that the information be free of errors, or, if errors are made, that they are corrected quickly.
Like “every child deserves a pony,” emotionally aspirational statements like “information [should be] be free of errors” are examples of rhetorical tools used to condition the reader that what whatever conclusions that follows, the reader should already be prepared to agree with them.
After all, what child doesn’t deserve a pony?
They follow that with:
Conclusion: A basic prerequisite for making informed policy decisions is accurate and reliable statistics, even during times of uncertainty. Our investigation revealed 25 instances of numerical or statistical errors made by the CDC. Our investigation suggests 1) the need for greater diligence in data collection and reporting, and 2) that the federal entity responsible for reporting health statistics should be firewalled from the entity setting policy due to concerns of real or perceived systematic bias in errors.
It is hard to describe that conclusion as anything but blatant agitprop. The paper’s authors knew three things when they wrote it. They knew:
They knew CDC does not generate the data. The data is generated at the state and county level. The CDC’s job is only to analyze and disseminate the data sent to the CDC, at the pleasure of the states and their counties. In particular it is not possible for the CDC to introduce “systemic bias in errors” given the obvious checks that each state can have on the CDC’s presentation of that state’s data.
For example, Florida could report 100 pediatric COVID deaths but if the CDC consistently says Florida has 110 pediatric COVID deaths, at some point Florida is going to notice and cry foul.They knew CDC does not “set policy.” Certainly not at the state level. Again, in the United States the states are sovereign with regard to health care, including things like licensing medical professionals and hospitals, setting data collection standards, deciding how a death certificate should be filled out, etc. That is the power and the responsibility of the states and the states alone, not the CDC.
They knew that public doesn't know any of this. That creates the opportunity to use propaganda about the CDC’s methods and motives to sow distrust of the CDC’s data in the public’s mind while also ascribing to the CDC fictional powers over that states that it simply does not have. This creates long lasting institutional damage that is nearly impossible to reverse in the short term and that eventually corrodes society irreversibly in the long term.
Again, given their advanced degrees and backgrounds in economics and health, it is not reasonable to believe that the authors of this paper were not aware that they were intentionally creating disinformation in the public’s mind.
We sought to compile errors that we previously identified in our real time observation of the COVID-19 pandemic, including, but not limited to, news sources we read or watched, ACIP meeting or transcripts or slides we observed, the CDC and CDC Director’s Twitter accounts, MMWR research, and errors brought to our attention by other observers.
Another example of attempt to frame the issue. Throughout the paper the authors chose to characterize each and every single point to which the authors object as an “error” on the CDC’s part. In at least two of their objections, the “error” is an obvious typographical error of utter irrelevance to public policy.
When a handful of typographical errors in a handful of reports from an agency that produces tens of thousands of reports a year are presented as examples of institutional malfeasance, that’s a sign that the presenters are not arguing in good faith.
In general the flavor of “error” that the authors identify are merely statistics reported by the CDC that the paper’s authors simply do not like. They are “errors” not because they are wrong but because they risk shattering the pervasive myth that COVID is not a serious disease, particularly in children.
It is telling that the authors characterize all of their identified errors as erring in one direction and one direction only — namely the over-reporting of COVID risks and danger. The way they do this is easy — they seek any instance in which the CDC reported a statistic that was not to their liking and record that statistic as a CDC “error.”
Twenty (80%) of these instances exaggerated the severity of the COVID-19 situation, 3 (12%) instances simultaneously exaggerated and downplayed the severity of the situation, one instance was neutral, but was false, nonetheless, and one instance exaggerated the risks of the COVID-19 vaccines.
At the same time they simply ignore any CDC statistic that goes in the opposite direction. Throughout the pandemic there have been numerous instances in which CDC has had to revise upward previously reported statistics yet none of those instances ever appear as a CDC “error” in the paper, giving the readers the impression that the CDC only errs to over-report COVID and never to under-report.
Case reports vs. Death Certificates
The CDC receives two different sets of data from the states pertaining to COVID. One are death certificates that the CDC enumerates as COVID deaths. The other are case reports that the CDC also enumerates as COVID deaths.
The two sets of data — death certificates and case reports — ostensibly report the same things (COVID cases and deaths) but their methodologies are different because they are intended for different purposes. Because the sets of data attempt to count the same thing (COVID deaths) but use different methods to count it is extremely unlikely that an enumeration of both datasets will produce identical counts. Hopefully they will be close but they will never be identical.
The paper’s authors know this yet nonetheless compare the results from each and in bad faith flatly declare any instance in which they disagree as another example of CDC malfeasance and the over-counting of COVID’s disease impact.
Case reports go into what the CDC calls its “Data Tracker.” State case reports are generally much more timely than death certificates and thus much more useful for real-time monitoring of the situation than death certificates and this is a large reason for their existence.
Death certificates go into something called the National Center for Health Statistics (NCHS) database. The problem with death certificates is that they are subject to significant reporting lag — often on the order of six months to a year for pediatric death certificates.
They are useless for understanding the current state and impact of the pandemic.
Death certificates, for reasons I went into in Part One of this, are also subject to significant undercounting — particularly for pediatric death certificates. Undercounting of causes of death on death certificates is again a very well known issue in the industry and predates the pandemic.
In fact, the CDC has numerous disclaimers regarding the inaccuracy of death certificates in ascertaining specific causes of deaths. Media reports such as this, this and this describe the effect.
The authors of the paper in question deliberately ignore it.
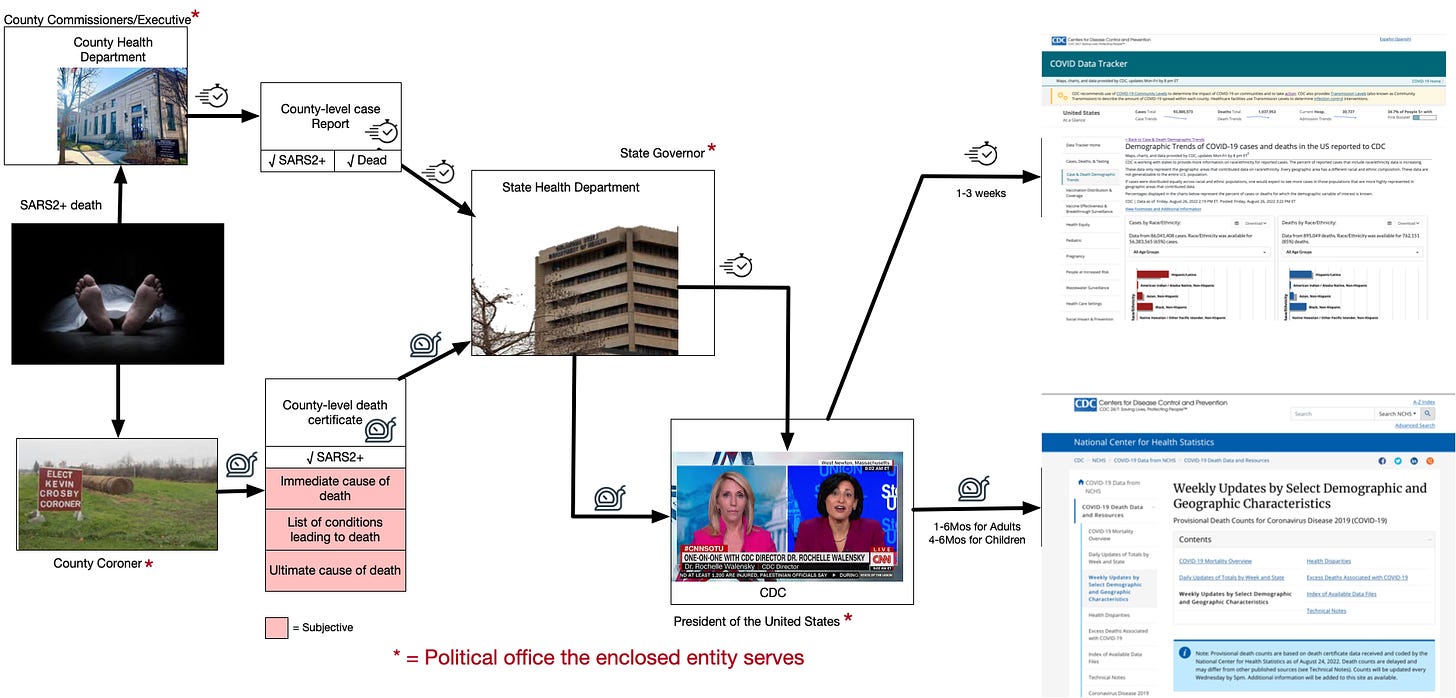
The NCHS numbers, derived from lagging death certificates, present a significant under count of the actual disease impact. Not just of COVID but of influenza, RSV, accidental deaths, etc. Again, the CDC has been quite clear for years why it does not use NCHS death certificate counts to ascertain the disease impact of influenza:
For a number of reasons, however, these surveillance systems do not capture every flu-related illness, medical visit, hospitalization or even death in the United States. CDC feels it is important to convey the full burden of seasonal flu and thus uses well-established scientific methods that have been reviewed by scientists outside of CDC to make comprehensive estimates of the total numbers of annual flu illnesses, flu-associated hospitalizations, and flu-associated deaths that occur each year.
Lest you think that not using death certificates is something unique for influenza, this is what the CDC said about why it does not use death certificates to count COVID cases:
The cumulative burden of COVID-19 is an estimate of the number of people who may have been infected, sick, hospitalized, or died as a result of a COVID-19 infection in the United States. Confirmed COVID-19 cases and deaths are nationally reported, but these cases and deaths likely represent only a fraction of the true number that have occurred in the population. COVID-19 infections, symptomatic illnesses, hospitalizations, and deaths might be underdetected and go unreported for a variety of reasons.
“Why CDC estimates COVID-19 Infections, Illnesses, Hospitalizations, and Deaths”
The CDC does not even count deaths from automobile accidents using the NCHS databases. Instead when asked how many people die in automobile accidents, the CDC defers to the National Highway Traffic Safety Administration, not NCHS death certificate counts.
Again, counting almost any disease by the number of times it appears as the cause of death on a death certificate is a surefire way to produce an undercount of the disease. The only reason to use NCHS death certificate counts as an “accurate” depiction of a disease’s burden is if one wishes to intentionally minimize the disease’s burden.
The paper’s authors know this, which is why they insist on using NCHS undercounts to characterize the disease impact of COVID — particularly the impact of COVID on children.
One of the paper’s authors, Kronhert, makes this explicit in a Substack post:
The CDC also has a system called CDC WONDER, where you can perform a search query for the number of deaths where Covid is listed only as the Underlying Cause of Death. This means the numbers from the CDC WONDER database are even lower, since they exclude deaths where Covid was only a contributing factor. WONDER updates monthly, and is due for an update any day now, so it’s a bit behind, but you can see it currently shows a little under 1000 pediatric deaths where Covid is listed as the Underlying Cause.
Here she uses NCHS death certificate data to come up with the number of pediatric COVID deaths (1,000) using the most restrictive count (underlying cause of death) — despite the warnings from the CDC of which she is well aware that NCHS counts are undercounts.
She then immediately pivots to say this:
For comparison, the CDC estimated there were 1,090 pediatric deaths from the H1N1 flu epidemic, from April - November 2009.
“The CDC's Flawed Data Tracker”
Did you catch that? Krohnert takes NCHS death certificate reports of COVID deaths, representing the greatest undercount, and compares them to CDC estimates of influenza deaths in one of the worst influenza seasons in two decades. And then uses that flawed comparison to conclude that, compared to flu, COVID is no big deal in children.
This is fundamentally dishonest. Krohnert knows that NCHS death certificate counts, particularly those using the most restrictive “underlying cause of death” (UCOD) criteria, undercount deaths.
In this proof-of-concept study, we assessed varying case definitions (suicide, alcohol-related, drug-related mortality using underlying cause of death [UCOD] versus multiple cause of death [MCOD]) … Alcohol use disorder codes accounted for 71% of additional alcohol-related deaths captured with the MCOD definition. Studies that rely on UCOD codes may be underestimating the burden of deaths
What if we go back and ask WONDER, the system that Kronhert used to come up with COVID deaths, to find out how many influenza deaths there were from April-November 2009? In other words, instead of making an apples (NCHS) to oranges (CDC estimates) comparison of COVID to flu, let’s make an apples (NCHS) to apples (NCHS, again) comparison:
The vast gulf between what is reported as being the death count from a disease (influenza in this case) and what the CDC estimates to be the actual number of deaths from a disease could not be made more clear.
According to Krohnert, the CDC estimated 1,090 pediatric deaths in one of the worst flu seasons in decades. Yet only 244 pediatric death certificates listed influenza as the cause of death in the same period — a difference of 1,090 / 244 = 4.5x
To drive the point home, let’s look at NCHS pediatric deaths from COVID in April-November 2022 for comparison:
208 times 4.5 is 936. If we compare the entire year of 2009 flu (307 deaths) to the entire year of COVID flu (438) deaths then we get 1,382 pediatric influenza deaths in 2009 to 1,971 pediatric COVID deaths in 2022 using the same NCHS-to-estimate multiplier (4.5x) for both.
And remember that 2009 was a record year for pediatric influenza deaths
The root of their complaint: NCHS counts don’t agree with case counts and that doesn’t fit our narrative (that it’s perfectly OK to purposefully infect children with a BSL-3 pathogen), therefore the CDC is wrong
The meat of the complaint in the paper is twofold:
The CDC uses state case numbers to report COVID deaths on its “Data Tracker,” not NCHS numbers
Deaths counted from case numbers (“Data Tracker”) are higher than deaths counted from NCHS numbers
And concludes for its readers from that that because case numbers are higher that the under-reported NCHS numbers — i.e. are numbers that the paper’s authors find inconvenient — that the CDC is reporting “statistical errors” and that COVID is actually not a serious pediatric disease.
Which is an allegation utterly without merit and unsupportable from the facts at hand. Again, over and over the CDC cautions that NCHS numbers — whether for flu or for COVID — are an undercount of the disease’s burden. Moreover on every CDC source that I could find cited in the paper there is an explicit disclaimer that the data provided is subject to change and revision as data comes in. Yet nowhere in the author’s paper do they acknowledge this.
If I tell the paper’s authors: “I think today’s high temperature will be about 80 degrees. But be careful as I measure the temperature outside because measuring the temperature inside will result in an undercount of how hot it really is. Oh, and that’s just my estimate. It could change"
Then it makes no sense whatsoever for them to come back and write “Statistical and numeric errors made by Greg during today’s temperature highs” saying that their thermometer, which is highly accurate, showed a different temperature when they measured the inside temperature and declared it the outside temperature.
Blatant propaganda
“Statistical and numerical errors made by the US Centers for Disease Control and Prevention During the COVID-19 Pandemic” is a blatant piece of political propaganda designed to undermine public health measures in the worst pandemic of over a century. COVID is a leading cause of death in every age group and has resulted in a sustained reduction of life expectancy in the United States, largely because of disinformation such as this paper.
Average life expectancy in a country is not driven by how long adults live. Average life expectancy in a country is driven by how many people die young.
The paper’s authors have a long and inglorious history of deliberate and willful distortion of facts during the pandemic. Jonathan Howard has documented many of them in his articles for Science Based Medicine as well as his book “We want them infected.”
At least three of the paper’s authors (Prasad, Krohnert, Hoeg) have long histories of being vehemently opposed to any form of protection against infection by the SARS2 pathogen in children. Instead advocating that the vascular BSL-3 pathogen should be allowed to rip freely through children as a way to create a prophylactic “Focused Infection” barrier of so-called “herd immunity,” using children as a permanent viral reservoir.
A reservoir from which infection can repeatedly spread while affording adults long-term protection — much like the mechanism of polio infections in children in accordance with the public health policy tenets of the “Great Barrington Declaration.”
Their paper is yet another form of support to their position that it is advantageous that children be repeatedly infected with the SARS-CoV-2 pathogen on the position that children are inherently immune to long-term harms from repeated SARS2 infections.
There is absolutely no scientific support for this position.
California, home to the institution (UCSF) with which Prasad and Haslam are affiliated has reported a cumulative 138 pediatric deaths from COVID as of this writing (March 28th). Georgia, the home state of Krohnert, reports 37 pediatric deaths and Florida, which employed Hoeg to argue for vaccination prohibitions in Florida’s children, has seen 122 pediatric deaths. These counts are all from the March 6th 2023 version of the CDC’s restricted state case database.
The total of pediatric deaths from COVID in just those three states is nearly three hundred. Three hundred children who are dead today and whose families grieve them who would almost certainly not be dead if they had access to and were given the SARS-CoV-2 vaccinations opposed by all four of the paper’s authors
Two of the paper’s authors (Prasad and Hoeg) are affiliated with the right-wing Brownstone Institute whose director, Jeffrey Tucker, was instrumental in the creation of the Great Barrington Declaration. Tucker is also affiliated with the Neo-confederate “League of the South” which credits him as one of the founders of the organization. Tucker has a long history of arguing against child labor laws.
The authors have a wide and influential following
Lest anyone be quick to discard their paper as a product of the reactionary fringe, it should be pointed out that the paper has been amplified and distributed wildly by individuals at the center of forming the US policy response to the pandemic.
For instance, Dr. Monica Gandhi forwarded links to the paper with the comment:
Dr. Gandhi is a frequent guest on mainstream media where she consistently downplays the impact of COVID, particularly in children, and argues for the removal of all pediatric protections such as masks and vaccinations.
This is not science. This is not medicine. This is just reactionary propaganda created to undermine public health responses to this and all future pandemics
The paper’s only purpose is to perpetuate the withholding of protection against illness and death in children from repeated SARS-CoV-2 infection for what can only be assumed are purely ideological and not scientific or medical justifications.
That is a crime that demands the immediate retraction of the paper. The authors should issue a formal apology and at least some of them, particularly Prasad, Hoeg and Haslam, should face consequences of institutional and professional sanction in accordance to the damage done by their reckless attacks on public health and their dereliction of their duty to extend all protections necessary to children to shield them from the harms of a deadly vascular pathogen.
One of the paper’s authors, Prasad, gave a hint when he wrote: “When democratically elected systems transform into totalitarian regimes, the transition is subtle, stepwise, and involves a combination of pre-planned as well as serendipitous events. Indeed, this was the case with Germany in the years 1929-1939, where Hitler was given a chance at governing” — implying that the pandemic was a hoax perpetrated for the purposes of imposing authoritarian dictatorships.
“BSL” stands for “Biosafetylevel.” Biosafety levels are divided into four levels with level one (1) being the most lenient with regard to required laboratory practices and level four being the most restrictive with regard to required laboratory practices. For example, influenza is a BSL-2 pathogen while Ebola is a BSL-4 pathogen. Examples of BSL-3 pathogens include tuberculosis and SARS-CoV-2